Falls are the leading cause of injury-related morbidity and mortality among older adults. In addition to fractures and traumatic brain injuries, ‘long lie’ is another serious consequence of falls in this population. Long lies are experienced by individuals who are unable to get up after a fall and remain on the ground for an extended period of time. Wearable sensor systems that can automatically detect falls and send an alert to care providers can reduce the frequency and severity of long lies. Ideally speaking, such fall detection systems/algorithms should be developed using real-life fall and activity data acquired directly from older adults as they go about their daily activities. However, recording falls through this approach could prove to be extremely time-consuming, spanning several years of strenuous data collection from a large population. While these efforts should be continued, designing and developing a comprehensive laboratory-based fall experiment representing a range of real-life falls and related activities in older adults can serve as an alternative to develop such fall detection algorithms. These algorithms can later be validated and easily adjusted on real-life data.
To design and carry out such a laboratory experiment with young adults, we first studied video footage of 227 falls experienced by 130 older residents of a local long-term care facility. Based on this footage, 86% of the falls were collectively due to (i) incorrect shift of bodyweight (41 %), (ii) tripping (21 %), (iii) hit/bump (11 %), (iv) collapse/loss of consciousness (10 %), and (v) slipping (3 %). Our experimental design included these falls, along with a range of near-falls and activities of daily living (ADLs). Near-falls are particularly important to be included in experiments in order to limit the occurrence of false positives.
Figure: Signals acquired from a waist-mounted tri-axial accelerometer from a typical participant during: A) falls, B) near-falls, and C) activities of daily living (ADLs). AP, ML and Inf/Sup denote acceleration in anterior/posterior, medial/lateral and inferior/superior directions.
On the aforementioned dataset, we tested the accuracy of five previously published threshold-based algorithms and five novel machine learning fall detection algorithms to differentiate falls from near-falls and ADLs. Our results show that the machine learning algorithms provided greater overall sensitivity and specificity than the threshold-based algorithms. Among the machine learning algorithms, Support Vector Machines provided the greatest sensitivity and specificity of 96% in distinguishing falls from non-falls. Our results provide a template for further improvement in designing a robust fall detection system, which is necessary for the development of ‘smart’ personal emergency response system that can automatically place a call for help in case of a fall to prevent long lies.
Publication
Aziz O, Musngi M, Park EJ, Mori G, Robinovitch SN. A comparison of accuracy of fall detection algorithms (threshold-based vs. machine learning) using waist-mounted tri-axial accelerometer signals from a comprehensive set of falls and non-fall trials. Med Biol Eng Comput. 2016 Apr 22. [Epub ahead of print] (http://www.ncbi.nlm.nih.gov/pubmed/27106749)
About the Author
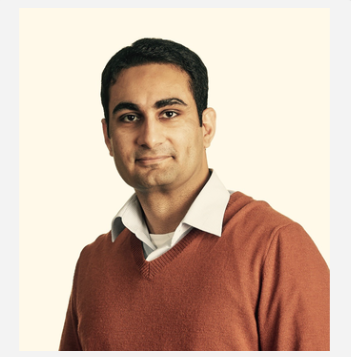
Omar Aziz
School of Engineering Science, Simon Fraser University
Omar Aziz received his Ph.D. degree in 2015 from the School of Engineering Science, Simon Fraser University (SFU), Burnaby, BC, Canada. He is a postdoctoral fellow at the Biomechatronic Systems Laboratory at SFU. His research interests include: machine learning, wearable sensors and their applications to human movement analysis, activity classification, injury prevention, biomechanics, robotics, and control.
Copyright
© 2018 by the author. Except as otherwise noted, the ISPGR blog, including its text and figures, is licensed under a Creative Commons Attribution-ShareAlike 4.0 International License. To view a copy of this license, visit https://creativecommons.org/licenses/by-sa/4.0/legalcode.
ISPGR blog (ISSN 2561-4703)
Are you interested in writing a blog post for the ISPGR website? If so, please email the ISGPR Secretariat with the following information:
- First and Last Name
- Institution/Affiliation
- Paper you will be referencing