Walking is one of the most fundamental human activities in daily life. Objective and accurate assessment of human gait provides valuable information to evaluate an individual’s gait pattern, diagnose gait abnormalities, and devise rehabilitation to restore or optimise the gait pattern. Currently, the majority of gait event detection methods are based on kinematic characteristics of trunk or foot movements, which can be obtained using inertial sensors, insoles with built-in pressure sensors or a combination of the above. All these devices have their own disadvantages. The inertial-based devices are accurate for detecting gait events during moderate and fast walking. However, their performance noticeably degrades at lower walking speeds, which is usually the pace for individuals with difficulty in walking. Foot switches and insole pressure sensors are not able to detect gait events during the swing phase, when the foot is not in contact with the floor.
Force myography (FMG) can be a promising solution for gait event detection. FMG is a muscle activity sensing technology that often utilizes an array of force pressure sensors surrounding a limb to register volumetric changes of the underlying musculotendinous complex during activity. FMG has recently gain interest, and has been investigated primarily for upper extremity gesture recognition and prosthesis control. The MENRVA lab (http://www.sfu.ca/menrva.html) has conducted a series of studies to explore the feasibility of a FMG ankle band for gait analysis, including detecting ankle movements, counting steps, and gait event detection. In this study, we used a FMG ankle band with an array of eight force sensing resistors (FSRs) on the ankle (Fig.1) to record the pressure changes during walking. Healthy young volunteers were recruited to walk slowly on a treadmill. A machine learning model was built on a part of the collected FMG data to detect gait events and partition gait phases. The preliminary results show that over 98.5% steps were correctly detected.
The current FMG ankle band is designed to accurately monitoring slow gait in senior people. We plan to further improve the performance of the strap and test the accuracy of this novel method to a wider population including stroke survivors, Parkinson’s disease, and multiple sclerosis. This technology is anticipated to be transferred to clinical settings and has the potential to improve the rehabilitation process and quality of life of those affected by gait impairments caused by various neurological conditions.
Figure: 1) The FSR (force sensing resistors) band for signal acquisition; 2) the strap placement on the ankle; and 3) the FMG (force myography) signals corresponding to detected gait phases. IC: Initial-Contact, MSt: Mid-Stance, PS: Pre-Swing, Sw: Swing.
Publication
K.H. Chu, X. Jiang, C. Menon, Wearable step counting using a force myography-based ankle strap, J. Rehabil. Assist. Technol. Eng. 4 (2017) 1–11. doi:10.1177/2055668317746307. URL
About the Author
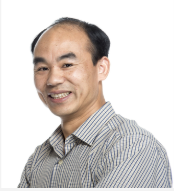
Xianta Jiang, Ph.D.
MENRVA Lab, Engineering Science, Simon Fraser University
Xianta Jiang is a Post-Doctoral Fellow in Engineering Science of Simon Fraser University (SFU), BC, Canada, working with Dr. Carlo Menon. He received his MSc from Zhejiang University in 1998 and his PhD from Simon Fraser University in 2015. His research interests include Human-Computer Interactions (HCI), Physiological Signals, Gait Analysis, Hand Gesture Recognition, Eye-tracking, Force Myography. He is an IEEE, ACM, and ISPGR member.
Copyright
© 2018 by the author. Except as otherwise noted, the ISPGR blog, including its text and figures, is licensed under a Creative Commons Attribution-ShareAlike 4.0 International License. To view a copy of this license, visit https://creativecommons.org/licenses/by-sa/4.0/legalcode.
ISPGR blog (ISSN 2561-4703)
Are you interested in writing a blog post for the ISPGR website? If so, please email the ISGPR Secretariat with the following information:
- First and Last Name
- Institution/Affiliation
- Paper you will be referencing